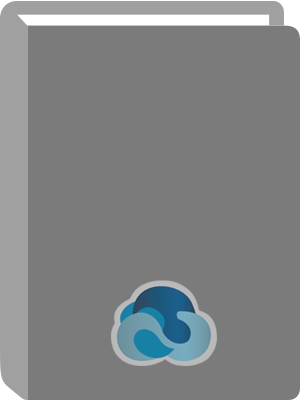
Basic econometrics
Title:
Basic econometrics
Author:
Gujarati, Damodar N.
ISBN:
9780070252141
9780071139632
9780072345759
9780071139649
Personal Author:
Edition:
3rd ed.
Publication Information:
New York : McGraw-Hill, ©1995.
Physical Description:
xxiii, 838 pages : illustrations ; 25 cm
General Note:
"International edition"--Title page verso.
Contents:
Single-Equation Regression Models -- The Nature of Regression Analysis Historical Origin of the Term "Regression" The Modern Interpretation of Regression Examples Statistical vs. Deterministic Relationships Regression vs. Causation Regression vs. Correlation Terminology and Notation The Nature and Sources of Data for Econometric Analysis Types of Data The Sources of Data The Accuracy of Data Exercises Appendix 1A Sources of Economic Data Sources of Financial Data Two-Variable Regression Analysis: Some Basic Ideas A Hypothetical Example The Concept of Population Regression Function (PRF) The Meaning of the Term "Linear" Linearity in the Variables Linearity in the Parameters Stochastic Specification of PRF The Significance of the Stochastic Disturbance Term The Sample Regression Function (SRF) Exercises Two-Variable Regression Model: The Problem of Estimation The Method of Ordinary Least Squares The Classical Linear Regression Model: The Assumptions Underlying the Method of Least Squares How Realistic Are These Assumptions? Precision or Standard Errors of Least-Squares Estimates Properties of Least-Squares Estimators: The Gauss-Markov Theorem The Coefficient of Determination r2: A Measure of "Goodness of Fit" A Numerical Example Illustrative Examples Coffee Consumption in the United States, 1970-1980 Keynesian Consumption Function for the United States, 1980-1991 Computer Output for the Coffee Demand Function A Note on Monte Carlo Experiments Exercises Problems Appendix 3A Derivation of Least-Squares Estimates Linearity and Unbiasedness Properties of Least-Squares Estimators Variances and Standard Errors of Least-Squares Estimators Covariance between B1 and B2 The Least-Squares Estimator of o2 Minimum-Variance Property of Least-Squares Estimators SAS Output of the Coffee Demand Function (3.7.1) The Normality Assumption: Classical Normal Linear Regression Model (CNLRM) The Probability Distribution of Disturbances ui The Normality Assumption Properties of OLS Estimators under the Normality Assumption The Method of Maximum Likelihood (ML) Probability Distributions Related to the Normal Distribution: The t, Chi-square (X2), and F Distributions Appendix 4A Maximum Likelihood Estimation of Two-Variable Regression Model Maximum Likelihood Estimation of the Consumption-Income Example Appendix 4A Exercises Two-Variable Regression: Interval Estimation and Hypothesis Testing Statistical Prerequisites Interval Estimation: Some Basic Ideas Confidence Intervals for Regression Coefficients B1 and B2 Confidence Interval for B2 Confidence Interval for B1 Confidence Interval for B1 and B2 Simultaneously Confidence Interval for o2 Hypothesis Testing: General Comments Hypothesis Testing: The Confidence-Interval Approach Two-Sided or Two-Tail Test One-Sided or One-Tail Test Hypothesis Testing: The Test-of-Significance Approach Testing the Significance of Regression Coefficients: The t-Test Testing the Significance of o2: the X2 Test Hypothesis Testing: Some Practical Aspects The Meaning of "Accepting" or "Rejecting" a Hypothesis The "Zero" Null Hypothesis and the "2-t" Rule of Thumb Forming the Null and Alternative Hypotheses Choosing a, the Level of Significance The Exact Level of Significance: The p Value Statistical Significance versus Practical Significance The Choice between Confidence-Interval and Test-of-Significance Approaches to Hypothesis Testing Regression Analysis and Analysis of Variance Application of Regression Analysis: The Problem of Prediction Mean Prediction Individual Prediction Reporting the Results of Regression Analysis Evaluating the Results of Regression Analysis Normality Test Other Tests of Model Adequacy Exercises Problems Appendix 5A Derivation of Equation (5.3.2) Derivation of Equation (5.9.1) Derivation of Equations (5.10.2) and (5.10.6) Variance of Mean Prediction Variance of Individual Prediction Extensions of the Two-Variable Linear Regression Model Regression through the Origin r2 for Regression-through-Origin Model An Illustrative Example: The Characteristic Line of Portfolio Theory Scaling and Units of Measurement A Numerical Example: The Relationship between GPDI and GNP, United States, 1974-1983 A Word about Interpretation Functional Forms of Regression Models How to Measure Elasticity: The Log-Linear Model An Illustrative Example: The Coffee Demand Function Revisited Semilog Models: Log-Lin and Lin-Log Models How to Measure the Growth Rate: The Log-Lin Model The Lin-Log Model Reciprocal Models An Illustrative Example: The Phillips Curve for the United Kingdom, 1950-1966 Summary of Functional Forms A Note on the Nature of the Stochastic Error Term: Additive versus Multiplicative Stochastic Error Term Exercises Problems Appendix 6A Derivation of Least-Squares Estimators for Regression through the Origin SAS Output of the Characteristic Line (6.1.12) SAS Output of the United Kingdom Phillips Curve Regression (6.6.2) Multiple Regression Analysis: The Problem of Estimation The Three-Variable Model: Notation and Assumptions Interpretation of Multiple Regression Equation The Meaning of Partial Regression Coefficients OLS and ML Estimation of the Partial Regression Coefficients OLS Estimators Variances and Standard Errors of OLS Estimators Properties of OLS Estimators Maximum Likelihood Estimators The Multiple Coefficient of Determination R2 and the Multiple Coefficient of Correlation R Example 7.1: The Expectations-Augmented Phillips Curve for the United States, 1970-1982 Simple Regression in the Context of Multiple Regression: Introduction to Specification Bias R2 and the Adjusted R2 Comparing Two R2 Values Example 7.2: Coffee Demand Function Revisited The "Game" of Maximizing R2 Partial Correlation Coefficients Explanation of Simple and Partial Correlation Coefficients Interpretation of Simple and Partial Correlation Coefficients Example 7.3: The Cobb-Douglas Production Function: More on Functional Form Polynomial Regression Models Example 7.4: Estimating the Total Cost Function Empirical Results Exercises Problems Appendix 7A Derivation of OLS Estimators Given in Equations (7.4.3) and (7.4.5) Equality between a1 of (7.3.5) and B2 of (7.4.7) Derivation of Equation (7.4.19) Maximum Likelihood Estimation of the Multiple Regression Model The Proof that E(b12) = B2 + B3b32 (Equation 7.7.4) SAS Output of the Expectations-Augmented Phillips Curve (7.6.2) SAS Output of the Cobb-Douglas Production Function (7.10.4) Multiple Regression Analysis: The Problem of Inference The Normality Assumption Once Again Example 8.1: U.S. Personal Consumption and Personal Disposal Income Relation, 1956-1970 Hypothesis Testing in Multiple Regression: General Comments Hypothesis Testing about Individual Partial Regression Coefficients Testing the Overall Significance of the Sample Regression The Analysis of Variance Approach to Testing the Overall Significance of an Observed Multiple Regression: The F Test An Important Relationship between R2 and F The "Incremental," or "Marginal," Contribution of an Explanatory Variable Testing the Equality of Two Regression Coefficients Example 8.2: The Cubic Cost Function Revisited Restricted Least Squares: Testing Linear Equality Restrictions The t Test Approach The F Test Approach: Restricted Least Squares Example 8.3: The Cobb-Douglas Production Function for Taiwanese Agricultural Sector, 1958-1972 General F Testing Comparing Two Regressions: Testing for Structural Stability of Regression Models Testing the Functional Form of Regression: Choosing between Linear and Log-Linear Regression Models Example 8.5: The Demand for Roses Prediction with Multiple Regression The Troika of Hypothesis Tests: The Likelihood Ratio (LR), Wald (W), and Lagrange Multiplier (LM) Tests The Road Ahead Exercises Problems Appendix 8A Likelihood Ratio (LR) Test The Matrix Approach to Linear Regression Model
Subject Term:
Electronic Access:
Table of contents http://catdir.loc.gov/catdir/enhancements/fy0602/94035295-t.htmlPublisher description http://catdir.loc.gov/catdir/enhancements/fy0602/94035295-d.html