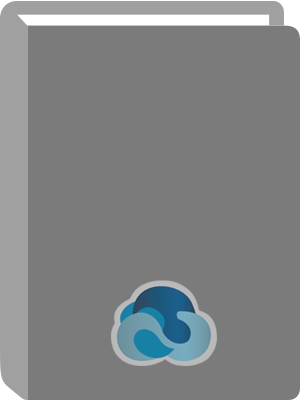
R and data mining : examples and case studies
Title:
R and data mining : examples and case studies
Author:
Zhao, Yanchang, 1977-
ISBN:
9780123969637
Personal Author:
Edition:
First edition.
Publication Information:
Amsterdam : Academic Press, an imprint of Elsevier, 2013.
Physical Description:
pages cm.
Contents:
Machine generated contents note: 1.Introduction -- 1.1.Data Mining -- 1.2.R -- 1.3.Datasets -- 1.3.1.The Iris Dataset -- 1.3.2.The Bodyfat Dataset -- 2.Data Import and Export -- 2.1.Save and Load R Data -- 2.2.Import from and Export to .CSV Files -- 2.3.Import Data from SAS -- 2.4.Import/Export via ODBC -- 2.4.1.Read from Databases -- 2.4.2.Output to and Input from EXCEL Files -- 3.Data Exploration -- 3.1.Have a Look at Data -- 3.2.Explore Individual Variables -- 3.3.Explore Multiple Variables -- 3.4.More Explorations -- 3.5.Save Charts into Files -- 4.Decision Trees and Random Forest -- 4.1.Decision Trees with Package party -- 4.2.Decision Trees with Package rpart -- 4.3.Random Forest -- 5.Regression -- 5.1.Linear Regression -- 5.2.Logistic Regression -- 5.3.Generalized Linear Regression -- 5.4.Non-Linear Regression -- 6.Clustering -- 6.1.The k-Means Clustering -- 6.2.The k-Medoids Clustering -- 6.3.Hierarchical Clustering -- 6.4.Density-Based Clustering --
Contents note continued: 7.Outlier Detection -- 7.1.Univariate Outlier Detection -- 7.2.Outlier Detection with LOF -- 7.3.Outlier Detection by Clustering -- 7.4.Outlier Detection from Time Series -- 7.5.Discussions -- 8.Time Series Analysis and Mining -- 8.1.Time Series Data in R -- 8.2.Time Series Decomposition -- 8.3.Time Series Forecasting -- 8.4.Time Series Clustering -- 8.4.1.Dynamic Time Warping -- 8.4.2.Synthetic Control Chart Time Series Data -- 8.4.3.Hierarchical Clustering with Euclidean Distance -- 8.4.4.Hierarchical Clustering with DTW Distance -- 8.5.Time Series Classification -- 8.5.1.Classification with Original Data -- 8.5.2.Classification with Extracted Features -- 8.5.3.k-NN Classification -- 8.6.Discussions -- 8.7.Further Readings -- 9.Association Rules -- 9.1.Basics of Association Rules -- 9.2.The Titanic Dataset -- 9.3.Association Rule Mining -- 9.4.Removing Redundancy -- 9.5.Interpreting Rules -- 9.6.Visualizing Association Rules --
Contents note continued: 9.7.Discussions and Further Readings -- 10.Text Mining -- 10.1.Retrieving Text from Twitter -- 10.2.Transforming Text -- 10.3.Stemming Words -- 10.4.Building a Term-Document Matrix -- 10.5.Frequent Terms and Associations -- 10.6.Word Cloud -- 10.7.Clustering Words -- 10.8.Clustering Tweets -- 10.8.1.Clustering Tweets with the k-Means Algorithm -- 10.8.2.Clustering Tweets with the k-Medoids Algorithm -- 10.9.Packages, Further Readings, and Discussions -- 11.Social Network Analysis -- 11.1.Network of Terms -- 11.2.Network of Tweets -- 11.3.Two-Mode Network -- 11.4.Discussions and Further Readings -- 12.Case Study I: Analysis and Forecasting of House Price Indices -- 12.1.Importing HPI Data -- 12.2.Exploration of HPI Data -- 12.3.Trend and Seasonal Components of HPI -- 12.4.HPI Forecasting -- 12.5.The Estimated Price of a Property -- 12.6.Discussion -- 13.Case Study II: Customer Response Prediction and Profit Optimization -- 13.1.Introduction --
Contents note continued: 13.2.The Data of KDD Cup 1998 -- 13.3.Data Exploration -- 13.4.Training Decision Trees -- 13.5.Model Evaluation -- 13.6.Selecting the Best Tree -- 13.7.Scoring -- 13.8.Discussions and Conclusions -- 14.Case Study III: Predictive Modeling of Big Data with Limited Memory -- 14.1.Introduction -- 14.2.Methodology -- 14.3.Data and Variables -- 14.4.Random Forest -- 14.5.Memory Issue -- 14.6.Train Models on Sample Data -- 14.7.Build Models with Selected Variables -- 14.8.Scoring -- 14.9.Print Rules -- 14.9.1.Print Rules in Text -- 14.9.2.Print Rules for Scoring with SAS -- 14.10.Conclusions and Discussion -- 15.Online Resources -- 15.1.R Reference Cards -- 15.2.R -- 15.3.Data Mining -- 15.4.Data Mining with R -- 15.5.Classification/Prediction with R -- 15.6.Time Series Analysis with R -- 15.7.Association Rule Mining with R -- 15.8.Spatial Data Analysis with R -- 15.9.Text Mining with R -- 15.10.Social Network Analysis with R --
Contents note continued: 15.11.Data Cleansing and Transformation with R -- 15.12.Big Data and Parallel Computing with R.
Added Author: